Supervised learning of few dirty bosons with variable particle number
Pere Mujal, Àlex Martínez Miguel, Artur Polls, Bruno Juliá-Díaz, Sebastiano Pilati
SciPost Phys. 10, 073 (2021) · published 24 March 2021
- doi: 10.21468/SciPostPhys.10.3.073
- Submissions/Reports
-
Abstract
We investigate the supervised machine learning of few interacting bosons in optical speckle disorder via artificial neural networks. The learning curve shows an approximately universal power-law scaling for different particle numbers and for different interaction strengths. We introduce a network architecture that can be trained and tested on heterogeneous datasets including different particle numbers. This network provides accurate predictions for all system sizes included in the training set and, by design, is suitable to attempt extrapolations to (computationally challenging) larger sizes. Notably, a novel transfer-learning strategy is implemented, whereby the learning of the larger systems is substantially accelerated and made consistently accurate by including in the training set many small-size instances.
Cited by 8
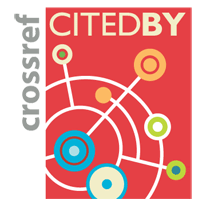
Authors / Affiliations: mappings to Contributors and Organizations
See all Organizations.- 1 2 3 Pere Mujal,
- 1 3 Àlex Martínez Miguel,
- 1 3 Artur Polls,
- 1 3 Bruno Juliá-Díaz,
- 4 Sebastiano Pilati
- 1 Universitat de Barcelona / University of Barcelona [UB]
- 2 Instituto de Física Interdisciplinar y Sistemas Complejos / Institute for Cross-Disciplinary Physics and Complex Systems [IFISC]
- 3 Institut de Ciènces del Cosmos / Institute of Cosmos Sciences, University of Barcelona [ICCUB]
- 4 Università degli Studi di Camerino / University of Camerino
- European Commission [EC]
- Generalitat de Catalunya / Government of Catalonia
- Ministero dell’Istruzione, dell’Università e della Ricerca (MIUR) (through Organization: Ministero dell'Istruzione, dell'Università e della Ricerca / Ministry of Education, Universities and Research [MIUR])