A supervised learning algorithm for interacting topological insulators based on local curvature
Paolo Molignini, Antonio Zegarra, Evert van Nieuwenburg, R. Chitra, Wei Chen
SciPost Phys. 11, 073 (2021) · published 28 September 2021
- doi: 10.21468/SciPostPhys.11.3.073
- Submissions/Reports
-
Abstract
Topological order in solid state systems is often calculated from the integration of an appropriate curvature function over the entire Brillouin zone. At topological phase transitions where the single particle spectral gap closes, the curvature function diverges and changes sign at certain high symmetry points in the Brillouin zone. These generic properties suggest the introduction of a supervised machine learning scheme that uses only the curvature function at the high symmetry points as input data. We apply this scheme to a variety of interacting topological insulators in different dimensions and symmetry classes. We demonstrate that an artificial neural network trained with the noninteracting data can accurately predict all topological phases in the interacting cases with very little numerical effort. Intriguingly, the method uncovers a ubiquitous interaction-induced topological quantum multicriticality in the examples studied.
Cited by 8
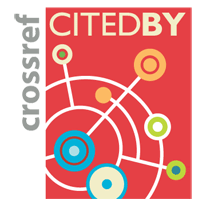
Authors / Affiliations: mappings to Contributors and Organizations
See all Organizations.- 1 Paolo Molignini,
- 2 Antonio Zegarra,
- 3 Everard van Nieuwenburg,
- 4 Ramasubramanian Chitra,
- 2 Wei Chen
- 1 University of Cambridge
- 2 Pontifícia Universidade Católica do Rio de Janeiro / Pontifical Catholic University of Rio de Janeiro [PUC-Rio]
- 3 Niels Bohr Institute [NBI]
- 4 Eidgenössische Technische Hochschule Zürich / Swiss Federal Institute of Technology in Zurich (ETH) [ETH Zurich]
- Engineering and Physical Sciences Research Council [EPSRC]
- Horizon 2020 (through Organization: European Commission [EC])