Towards a method to anticipate dark matter signals with deep learning at the LHC
Ernesto Arganda, Anibal D. Medina, Andres D. Perez, Alejandro Szynkman
SciPost Phys. 12, 063 (2022) · published 16 February 2022
- doi: 10.21468/SciPostPhys.12.2.063
- Submissions/Reports
-
Abstract
We study several simplified dark matter (DM) models and their signatures at the LHC using neural networks. We focus on the usual monojet plus missing transverse energy channel, but to train the algorithms we organize the data in 2D histograms instead of event-by-event arrays. This results in a large performance boost to distinguish between standard model (SM) only and SM plus new physics signals. We use the kinematic monojet features as input data which allow us to describe families of models with a single data sample. We found that the neural network performance does not depend on the simulated number of background events if they are presented as a function of $S/\sqrt{B}$, where $S$ and $B$ are the number of signal and background events per histogram, respectively. This provides flexibility to the method, since testing a particular model in that case only requires knowing the new physics monojet cross section. Furthermore, we also discuss the network performance under incorrect assumptions about the true DM nature. Finally, we propose multimodel classifiers to search and identify new signals in a more general way, for the next LHC run.
Cited by 5
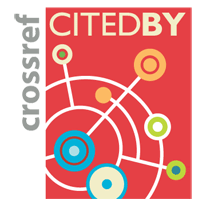
Authors / Affiliations: mappings to Contributors and Organizations
See all Organizations.- 1 2 Ernesto Arganda,
- 1 Anibal D. Medina,
- 1 Andres Daniel Perez,
- 1 Alejandro Szynkman
- 1 Universidad Nacional de La Plata / National University of La Plata [UNLP]
- 2 Instituto de Física Teórica / Institute for Theoretical Physics [IFT]
- Comunidad de Madrid
- Consejo Nacional de Investigaciones Cientificas y Tecnicas (CONICET)
- Secretaría de Estado de Investigacion, Desarrollo e Innovacion (through Organization: Ministerio de Economía, Industria y Competitividad / Ministry of Economy, Industry and Competitiveness [MINECO])