Role of stochastic noise and generalization error in the time propagation of neural-network quantum states
Damian Hofmann, Giammarco Fabiani, Johan H. Mentink, Giuseppe Carleo, Michael A. Sentef
SciPost Phys. 12, 165 (2022) · published 18 May 2022
- doi: 10.21468/SciPostPhys.12.5.165
- Submissions/Reports
-
Abstract
Neural-network quantum states (NQS) have been shown to be a suitable variational ansatz to simulate out-of-equilibrium dynamics in two-dimensional systems using time-dependent variational Monte Carlo (t-VMC). In particular, stable and accurate time propagation over long time scales has been observed in the square-lattice Heisenberg model using the Restricted Boltzmann machine architecture. However, achieving similar performance in other systems has proven to be more challenging. In this article, we focus on the two-leg Heisenberg ladder driven out of equilibrium by a pulsed excitation as a benchmark system. We demonstrate that unmitigated noise is strongly amplified by the nonlinear equations of motion for the network parameters, which causes numerical instabilities in the time evolution. As a consequence, the achievable accuracy of the simulated dynamics is a result of the interplay between network expressiveness and measures required to remedy these instabilities. We show that stability can be greatly improved by appropriate choice of regularization. This is particularly useful as tuning of the regularization typically imposes no additional computational cost. Inspired by machine learning practice, we propose a validation-set based diagnostic tool to help determining optimal regularization hyperparameters for t-VMC based propagation schemes. For our benchmark, we show that stable and accurate time propagation can be achieved in regimes of sufficiently regularized variational dynamics.
Cited by 10
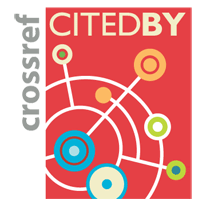
Authors / Affiliations: mappings to Contributors and Organizations
See all Organizations.- 1 Max-Planck-Institut für Struktur und Dynamik der Materie / Max Planck Institute for the Structure and Dynamics of Matter [MPSD]
- 2 Radboud Universiteit Nijmegen / Radboud University Nijmegen [RUN]
- 3 École Polytechnique Fédérale de Lausanne [EPFL]
- Deutsche Forschungsgemeinschaft / German Research FoundationDeutsche Forschungsgemeinschaft [DFG]
- European Research Council [ERC]
- Nederlandse Organisatie voor Wetenschappelijk Onderzoek / Netherlands Organisation for Scientific Research [NWO]
- Simons Foundation
- Stichting voor Fundamenteel Onderzoek der Materie / Foundation For Fundamental Research On Matter [FOM]