How to GAN Higher Jet Resolution
Pierre Baldi, Lukas Blecher, Anja Butter, Julian Collado, Jessica N. Howard, Fabian Keilbach, Tilman Plehn, Gregor Kasieczka, Daniel Whiteson
SciPost Phys. 13, 064 (2022) · published 23 September 2022
- doi: 10.21468/SciPostPhys.13.3.064
- Submissions/Reports
-
Abstract
QCD-jets at the LHC are described by simple physics principles. We show how super-resolution generative networks can learn the underlying structures and use them to improve the resolution of jet images. We test this approach on massless QCD-jets and on fat top-jets and find that the network reproduces their main features even without training on pure samples. In addition, we show how a slim network architecture can be constructed once we have control of the full network performance.
Cited by 7
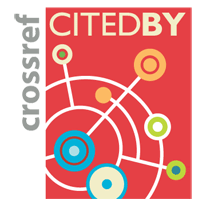
Authors / Affiliations: mappings to Contributors and Organizations
See all Organizations.- 1 Pierre Baldi,
- 2 Lukas Blecher,
- 2 Anja Butter,
- 1 Julian Collado,
- 1 Jessica N. Howard,
- 2 Fabian Keilbach,
- 2 Tilman Plehn,
- 3 Gregor Kasieczka,
- 1 Daniel Whiteson
- 1 University of California, Irvine [UCI]
- 2 Ruprecht-Karls-Universität Heidelberg / Heidelberg University
- 3 Universität Hamburg / University of Hamburg [UH]
Funders for the research work leading to this publication