Neural network approach to reconstructing spectral functions and complex poles of confined particles
Thibault Lechien, David Dudal
SciPost Phys. 13, 097 (2022) · published 13 October 2022
- doi: 10.21468/SciPostPhys.13.4.097
- Submissions/Reports
-
Abstract
Reconstructing spectral functions from propagator data is difficult as solving the analytic continuation problem or applying an inverse integral transformation are ill-conditioned problems. Recent work has proposed using neural networks to solve this problem and has shown promising results, either matching or improving upon the performance of other methods. We generalize this approach by not only reconstructing spectral functions, but also (possible) pairs of complex poles or an infrared (IR) cutoff. We train our network on physically motivated toy functions, examine the reconstruction accuracy and check its robustness to noise. Encouraging results are found on both toy functions and genuine lattice QCD data for the gluon propagator, suggesting that this approach may lead to significant improvements over current state-of-the-art methods.
Cited by 9
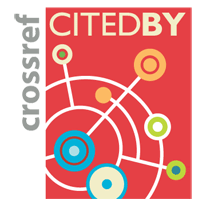
Authors / Affiliations: mappings to Contributors and Organizations
See all Organizations.- 1 Thibault Lechien,
- 1 2 David Dudal