Parameter-parallel distributed variational quantum algorithm
Yun-Fei Niu, Shuo Zhang, Chen Ding, Wan-Su Bao, He-Liang Huang
SciPost Phys. 14, 132 (2023) · published 26 May 2023
- doi: 10.21468/SciPostPhys.14.5.132
- Submissions/Reports
-
Abstract
Variational quantum algorithms (VQAs) have emerged as a promising near-term technique to explore practical quantum advantage on noisy intermediate-scale quantum (NISQ) devices. However, the inefficient parameter training process due to the incompatibility with backpropagation and the cost of a large number of measurements, posing a great challenge to the large-scale development of VQAs. Here, we propose a parameter-parallel distributed variational quantum algorithm (PPD-VQA), to accelerate the training process by parameter-parallel training with multiple quantum processors. To maintain the high performance of PPD-VQA in the realistic noise scenarios, a alternate training strategy is proposed to alleviate the acceleration attenuation caused by noise differences among multiple quantum processors, which is an unavoidable common problem of distributed VQA. Besides, the gradient compression is also employed to overcome the potential communication bottlenecks. The achieved results suggest that the PPD-VQA could provide a practical solution for coordinating multiple quantum processors to handle large-scale real-word applications.
Cited by 3
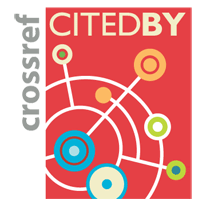
Authors / Affiliations: mappings to Contributors and Organizations
See all Organizations.- 1 Yun-Fei Niu,
- 1 Shuo Zhang,
- 1 Chen Ding,
- 1 Wan-Su Bao,
- 1 2 He-Liang Huang
- 1 Henan Key Laboratory of Quantum Information and Cryptography
- 2 中国科学技术大学 / University of Science and Technology of China [USTC]