MadNIS - Neural multi-channel importance sampling
Theo Heimel, Ramon Winterhalder, Anja Butter, Joshua Isaacson, Claudius Krause, Fabio Maltoni, Olivier Mattelaer, Tilman Plehn
SciPost Phys. 15, 141 (2023) · published 6 October 2023
- doi: 10.21468/SciPostPhys.15.4.141
- Submissions/Reports
-
Abstract
Theory predictions for the LHC require precise numerical phase-space integration and generation of unweighted events. We combine machine-learned multi-channel weights with a normalizing flow for importance sampling, to improve classical methods for numerical integration. We develop an efficient bi-directional setup based on an invertible network, combining online and buffered training for potentially expensive integrands. We illustrate our method for the Drell-Yan process with an additional narrow resonance.
Cited by 3
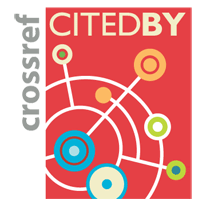
Authors / Affiliations: mappings to Contributors and Organizations
See all Organizations.- 1 Theo Heimel,
- 2 Ramon Winterhalder,
- 1 3 Anja Butter,
- 4 Joshua Isaacson,
- 1 Claudius Krause,
- 2 5 Fabio Maltoni,
- 2 Olivier Mattelaer,
- 1 Tilman Plehn
- 1 Ruprecht-Karls-Universität Heidelberg / Heidelberg University
- 2 Université catholique de Louvain [UCL]
- 3 Sorbonne Université / Sorbonne University
- 4 Fermi National Accelerator Laboratory [Fermilab]
- 5 Università di Bologna / University of Bologna [UNIBO]
Funders for the research work leading to this publication
- Baden-Württemberg Stiftung
- Bundesministerium für Bildung und Forschung / Federal Ministry of Education and Research [BMBF]
- Carl-Zeiss-Stiftung / Carl Zeiss Foundation
- Deutsche Forschungsgemeinschaft / German Research FoundationDeutsche Forschungsgemeinschaft [DFG]
- Fermilab
- Fonds De La Recherche Scientifique - FNRS (FNRS) (through Organization: Fonds National de la Recherche Scientifique [FNRS])
- United States Department of Energy [DOE]
- Université Catholique de Louvain
- Waalse Gewest