Deep-learned Top Tagging with a Lorentz Layer
Anja Butter, Gregor Kasieczka, Tilman Plehn, Michael Russell
SciPost Phys. 5, 028 (2018) · published 26 September 2018
- doi: 10.21468/SciPostPhys.5.3.028
- Submissions/Reports
-
Abstract
We introduce a new and highly efficient tagger for hadronically decaying top quarks, based on a deep neural network working with Lorentz vectors and the Minkowski metric. With its novel machine learning setup and architecture it allows us to identify boosted top quarks not only from calorimeter towers, but also including tracking information. We show how the performance of our tagger compares with QCD-inspired and image-recognition approaches and find that it significantly increases the performance for strongly boosted top quarks.
TY - JOUR
PB - SciPost Foundation
DO - 10.21468/SciPostPhys.5.3.028
TI - Deep-learned Top Tagging with a Lorentz Layer
PY - 2018/09/26
UR - https://www.scipost.org/SciPostPhys.5.3.028
JF - SciPost Physics
JA - SciPost Phys.
VL - 5
IS - 3
SP - 028
A1 - Butter, Anja
AU - Kasieczka, Gregor
AU - Plehn, Tilman
AU - Russell, Michael
AB - We introduce a new and highly efficient tagger for hadronically decaying top quarks, based on a deep neural network working with Lorentz vectors and the Minkowski metric. With its novel machine learning setup and architecture it allows us to identify boosted top quarks not only from calorimeter towers, but also including tracking information. We show how the performance of our tagger compares with QCD-inspired and image-recognition approaches and find that it significantly increases the performance for strongly boosted top quarks.
ER -
@Article{10.21468/SciPostPhys.5.3.028,
title={{Deep-learned Top Tagging with a Lorentz Layer}},
author={Anja Butter and Gregor Kasieczka and Tilman Plehn and Michael Russell},
journal={SciPost Phys.},
volume={5},
pages={028},
year={2018},
publisher={SciPost},
doi={10.21468/SciPostPhys.5.3.028},
url={https://scipost.org/10.21468/SciPostPhys.5.3.028},
}
Cited by 115
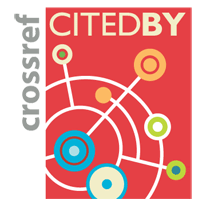
-
Lin et al., Boosting $$ H\to b\overline{b} $$ with machine learning
J. High Energ. Phys. 2018, 101 (2018) [Crossref] -
Kasieczka et al., Automating the ABCD method with machine learning
Phys. Rev. D 103, 035021 (2021) [Crossref] -
Macaluso et al., Pulling out all the tops with computer vision and deep learning
J. High Energ. Phys. 2018, 121 (2018) [Crossref] -
Alvarez et al., Exploring unsupervised top tagging using Bayesian inference
SciPost Phys. Core 6, 046 (2023) [Crossref] -
Collins et al., Extending the search for new resonances with machine learning
Phys. Rev. D 99, 014038 (2019) [Crossref] -
Konar et al., Energy-weighted message passing: an infra-red and collinear safe graph neural network algorithm
J. High Energ. Phys. 2022, 60 (2022) [Crossref] -
Han et al., Six top messages of new physics at the LHC
J. High Energ. Phys. 2019, 8 (2019) [Crossref] -
Tan et al., Machine learning phases and criticalities without using real data for training
Phys. Rev. B 102, 224434 (2020) [Crossref] -
Bernreuther et al., Casting a graph net to catch dark showers
SciPost Phys. 10, 046 (2021) [Crossref] -
Heimel et al., QCD or what?
SciPost Phys. 6, 030 (2019) [Crossref] -
Lester et al., Lorentz and permutation invariants of particles III: Constraining nonstandard sources of parity violation
Int. J. Mod. Phys. A 37, 2250093 (2022) [Crossref] -
Kasieczka et al., Quark-gluon tagging: Machine learning vs detector
SciPost Phys. 6, 069 (2019) [Crossref] -
D’Agnolo et al., Learning new physics from a machine
Phys. Rev. D 99, 015014 (2019) [Crossref] -
Shlomi et al., Graph neural networks in particle physics
Mach. Learn.: Sci. Technol. 2, 021001 (2021) [Crossref] -
Araz et al., Combine and conquer: event reconstruction with Bayesian Ensemble Neural Networks
J. High Energ. Phys. 2021, 296 (2021) [Crossref] -
Dreyer et al., Jet tagging in the Lund plane with graph networks
J. High Energ. Phys. 2021, 52 (2021) [Crossref] -
Dasgupta et al., Investigating top tagging with Ym-Splitter and N-subjettiness
J. High Energ. Phys. 2021, 92 (2021) [Crossref] -
Dillon et al., A normalized autoencoder for LHC triggers
SciPost Phys. Core 6, 074 (2023) [Crossref] -
Bogatskiy et al., Explainable equivariant neural networks for particle physics: PELICAN
J. High Energ. Phys. 2024, 113 (2024) [Crossref] -
Mondal et al., Machine learning in high energy physics: a review of heavy-flavor jet tagging at the LHC
Eur. Phys. J. Spec. Top., (2024) [Crossref] -
Nachman, A guide for deploying Deep Learning in LHC searches: How to achieve optimality and account for uncertainty
SciPost Phys. 8, 090 (2020) [Crossref] -
Louppe et al., QCD-aware recursive neural networks for jet physics
J. High Energ. Phys. 2019, 57 (2019) [Crossref] -
Li et al., Tag Z boson jets via convolutional neural networks
Acta Phys. Sin. 70, 061301 (2021) [Crossref] -
Larkoski et al., Jet substructure at the Large Hadron Collider: A review of recent advances in theory and machine learning
Physics Reports 841, 1 (2020) [Crossref] -
Biscarat et al., Jet Single Shot Detection
EPJ Web Conf. 251, 04027 (2021) [Crossref] -
Badea et al., Solving combinatorial problems at particle colliders using machine learning
Phys. Rev. D 106, 016001 (2022) [Crossref] -
Gong et al., An efficient Lorentz equivariant graph neural network for jet tagging
J. High Energ. Phys. 2022, 30 (2022) [Crossref] -
Biscarat et al., End-to-End Jet Classification of Boosted Top Quarks with CMS Open Data
EPJ Web Conf. 251, 04030 (2021) [Crossref] -
Ba et al., SHAPER: can you hear the shape of a jet?
J. High Energ. Phys. 2023, 195 (2023) [Crossref] -
Moreno et al., Interaction networks for the identification of boosted
H→bb¯
decays
Phys. Rev. D 102, 012010 (2020) [Crossref] -
Dolan et al., Equivariant energy flow networks for jet tagging
Phys. Rev. D 103, 074022 (2021) [Crossref] -
Choi et al., Infrared safety of a neural-net top tagging algorithm
J. High Energ. Phys. 2019, 132 (2019) [Crossref] -
Erdmann et al., Maximum performance of strange-jet tagging at hadron colliders
J. Inst. 16, P08039 (2021) [Crossref] -
Doglioni et al., Physics Inspired Deep Neural Networks for Top Quark Reconstruction
EPJ Web Conf. 245, 06029 (2020) [Crossref] -
Luo et al., Quark jet versus gluon jet: fully-connected neural networks with high-level features
Sci. China Phys. Mech. Astron. 62, 991011 (2019) [Crossref] -
Leigh et al., PC-JeDi: Diffusion for particle cloud generation in high energy physics
SciPost Phys. 16, 018 (2024) [Crossref] -
Brauer et al.,
, 1437 (2022) [Crossref] -
Moreno et al., JEDI-net: a jet identification algorithm based on interaction networks
Eur. Phys. J. C 80, 58 (2020) [Crossref] -
Kasieczka et al., Per-object systematics using deep-learned calibration
SciPost Phys. 9, 089 (2020) [Crossref] -
Shen, A Transdisciplinary Review of Deep Learning Research and Its Relevance for Water Resources Scientists
Water Resources Research 54, 8558 (2018) [Crossref] -
Onyisi et al., Comparing point cloud strategies for collider event classification
Phys. Rev. D 108, 012001 (2023) [Crossref] -
Andrews et al., End-to-end jet classification of boosted top quarks with the CMS open data
Phys. Rev. D 105, 052008 (2022) [Crossref] -
Erdmann et al., Reinforced sorting networks for particle physics analyses
J. Phys.: Conf. Ser. 1525, 012098 (2020) [Crossref] -
Batson et al., Topological obstructions to autoencoding
J. High Energ. Phys. 2021, 280 (2021) [Crossref] -
Anisha et al., BSM reach of four-top production at the LHC
Phys. Rev. D 108, 035001 (2023) [Crossref] -
Bradshaw et al., Mass agnostic jet taggers
SciPost Phys. 8, 011 (2020) [Crossref] -
Baldi et al., How to GAN Higher Jet Resolution
SciPost Phys. 13, 064 (2022) [Crossref] -
Chen et al., Deep learning jet substructure from two-particle correlations
Phys. Rev. D 101, 114025 (2020) [Crossref] -
Moore et al., Reports of my demise are greatly exaggerated: $N$-subjettiness taggers take on jet images
SciPost Phys. 7, 036 (2019) [Crossref] -
Monaco et al., Theory-Guided Deep Learning Algorithms: An Experimental Evaluation
Electronics 11, 2850 (2022) [Crossref] -
Forestano et al., Deep learning symmetries and their Lie groups, algebras, and subalgebras from first principles
Mach. Learn.: Sci. Technol. 4, 025027 (2023) [Crossref] -
Komiske et al., Energy flow networks: deep sets for particle jets
J. High Energ. Phys. 2019, 121 (2019) [Crossref] -
Murnane et al., Semi-Equivariant GNN Architectures for Jet Tagging
J. Phys.: Conf. Ser. 2438, 012121 (2023) [Crossref] -
Monaco et al.,
1652, 256 (2022) [Crossref] -
Park et al., Quasi anomalous knowledge: searching for new physics with embedded knowledge
J. High Energ. Phys. 2021, 30 (2021) [Crossref] -
Albouy et al., Theory, phenomenology, and experimental avenues for dark showers: a Snowmass 2021 report
Eur. Phys. J. C 82, 1132 (2022) [Crossref] -
Fraser et al., Jet charge and machine learning
J. High Energ. Phys. 2018, 93 (2018) [Crossref] -
D’Agnolo et al., Learning multivariate new physics
Eur. Phys. J. C 81, 89 (2021) [Crossref] -
Butter et al., Performance versus resilience in modern quark-gluon tagging
SciPost Phys. Core 6, 085 (2023) [Crossref] -
Bollweg et al., Deep-learning jets with uncertainties and more
SciPost Phys. 8, 006 (2020) [Crossref] -
Andreassen et al., binary junipr: An Interpretable Probabilistic Model for Discrimination
Phys. Rev. Lett. 123, 182001 (2019) [Crossref] -
Atkinson et al., IRC-Safe Graph Autoencoder for Unsupervised Anomaly Detection
Front. Artif. Intell. 5, 943135 (2022) [Crossref] -
Komiske et al., An operational definition of quark and gluon jets
J. High Energ. Phys. 2018, 59 (2018) [Crossref] -
Abdughani et al., Supervised Deep Learning in High Energy Phenomenology: a Mini Review*
Commun. Theor. Phys. 71, 955 (2019) [Crossref] -
Piscopo et al., Solving differential equations with neural networks: Applications to the calculation of cosmological phase transitions
Phys. Rev. D 100, 016002 (2019) [Crossref] -
Ju et al., Supervised jet clustering with graph neural networks for Lorentz boosted bosons
Phys. Rev. D 102, 075014 (2020) [Crossref] -
Datta et al., Automating the construction of jet observables with machine learning
Phys. Rev. D 100, 095016 (2019) [Crossref] -
Chakraborty et al., Interpretable deep learning for two-prong jet classification with jet spectra
J. High Energ. Phys. 2019, 135 (2019) [Crossref] -
Pol et al., Lightweight jet reconstruction and identification as an object detection task
Mach. Learn.: Sci. Technol. 3, 025016 (2022) [Crossref] -
Amram et al., Tag N’ Train: a technique to train improved classifiers on unlabeled data
J. High Energ. Phys. 2021, 153 (2021) [Crossref] -
Andrews,
, 59 (2023) [Crossref] -
Kar et al., Exploring jet substructure in semi-visible jets
SciPost Phys. 10, 084 (2021) [Crossref] -
Benato et al., Teaching machine learning with an application in collider particle physics
J. Inst. 15, C09011 (2020) [Crossref] -
Bieringer et al., Measuring QCD Splittings with Invertible Networks
SciPost Phys. 10, 126 (2021) [Crossref] -
Hajer et al., Novelty detection meets collider physics
Phys. Rev. D 101, 076015 (2020) [Crossref] -
Nguyen et al., Topology Classification with Deep Learning to Improve Real-Time Event Selection at the LHC
Comput Softw Big Sci 3, 12 (2019) [Crossref] -
Chen et al., Boosted
W
and
Z
tagging with jet charge and deep learning
Phys. Rev. D 101, 053001 (2020) [Crossref] -
Kasieczka et al., The Machine Learning landscape of top taggers
SciPost Phys. 7, 014 (2019) [Crossref] -
Duarte et al., FPGA-Accelerated Machine Learning Inference as a Service for Particle Physics Computing
Comput Softw Big Sci 3, 13 (2019) [Crossref] -
Aaboud et al., Performance of top-quark and
$$\varvec{W}$$
W
-boson tagging with ATLAS in Run 2 of the LHC
Eur. Phys. J. C 79, 375 (2019) [Crossref] -
Chatterjee et al., Jets with electrons from boosted top quarks
J. High Energ. Phys. 2020, 170 (2020) [Crossref] -
Chiang et al., Vector boson fusion versus gluon-gluon fusion Higgs boson production with full-event deep learning: Toward a decay-agnostic tagger
Phys. Rev. D 107, 016014 (2023) [Crossref] -
von Rueden et al., Informed Machine Learning - A Taxonomy and Survey of Integrating Prior Knowledge into Learning Systems
IEEE Trans. Knowl. Data Eng., 1 (2021) [Crossref] -
Kasieczka et al., Robust Jet Classifiers through Distance Correlation
Phys. Rev. Lett. 125, 122001 (2020) [Crossref] -
Andreassen et al., Neural networks for full phase-space reweighting and parameter tuning
Phys. Rev. D 101, 091901 (2020) [Crossref] -
Bedeschi et al., Jet flavour tagging for future colliders with fast simulation
Eur. Phys. J. C 82, 646 (2022) [Crossref] -
Tumasyan et al., Reconstruction of decays to merged photons using end-to-end deep learning with domain continuation in the CMS detector
Phys. Rev. D 108, 052002 (2023) [Crossref] -
Chakraborty et al., Neural network-based top tagger with two-point energy correlations and geometry of soft emissions
J. High Energ. Phys. 2020, 111 (2020) [Crossref] -
Dillon et al., Symmetries, safety, and self-supervision
SciPost Phys. 12, 188 (2022) [Crossref] -
Tan et al., A universal neural network for learning phases
Eur. Phys. J. Plus 136, 1116 (2021) [Crossref] -
Alipour-fard et al., Pileup and Infrared Radiation Annihilation (PIRANHA): a paradigm for continuous jet grooming
J. High Energ. Phys. 2023, 157 (2023) [Crossref] -
Das et al., Feature selection with distance correlation
Phys. Rev. D 109, 054009 (2024) [Crossref] -
Hammad et al., Streamlined jet tagging network assisted by jet prong structure
J. High Energ. Phys. 2024, 176 (2024) [Crossref] -
Erdmann et al., Lorentz Boost Networks: autonomous physics-inspired feature engineering
J. Inst. 14, P06006 (2019) [Crossref] -
Hao et al., Lorentz group equivariant autoencoders
Eur. Phys. J. C 83, 485 (2023) [Crossref] -
Qu et al., Jet tagging via particle clouds
Phys. Rev. D 101, 056019 (2020) [Crossref] -
Dreyer et al., Leveraging universality of jet taggers through transfer learning
Eur. Phys. J. C 82, 564 (2022) [Crossref] -
Abdughani et al., Probing stop pair production at the LHC with graph neural networks
J. High Energ. Phys. 2019, 55 (2019) [Crossref] -
Blance et al., Adversarially-trained autoencoders for robust unsupervised new physics searches
J. High Energ. Phys. 2019, 47 (2019) [Crossref] -
Guo et al., Deep learning for
R
-parity violating supersymmetry searches at the LHC
Phys. Rev. D 98, 076017 (2018) [Crossref] -
Farina et al., Searching for new physics with deep autoencoders
Phys. Rev. D 101, 075021 (2020) [Crossref] -
Khot et al., A detailed study of interpretability of deep neural network based top taggers
Mach. Learn.: Sci. Technol. 4, 035003 (2023) [Crossref] -
Dillon et al., Uncovering latent jet substructure
Phys. Rev. D 100, 056002 (2019) [Crossref] -
Finke et al., Autoencoders for unsupervised anomaly detection in high energy physics
J. High Energ. Phys. 2021, 161 (2021) [Crossref] -
Gripaios et al., Lorentz- and permutation-invariants of particles
J. Phys. A: Math. Theor. 54, 155201 (2021) [Crossref] -
Alves et al., Towards recognizing the light facet of the Higgs boson
Mach. Learn.: Sci. Technol. 1, 045025 (2020) [Crossref] -
Ngairangbam et al., Invisible Higgs search through vector boson fusion: a deep learning approach
Eur. Phys. J. C 80, 1055 (2020) [Crossref] -
Roman et al., Oracle-Preserving Latent Flows
Symmetry 15, 1352 (2023) [Crossref] -
Bothmann et al., Reweighting a parton shower using a neural network: the final-state case
J. High Energ. Phys. 2019, 33 (2019) [Crossref] -
Benato et al., Shared Data and Algorithms for Deep Learning in Fundamental Physics
Comput Softw Big Sci 6, 9 (2022) [Crossref] -
Carrazza et al., Jet grooming through reinforcement learning
Phys. Rev. D 100, 014014 (2019) [Crossref] -
Li et al., Does Lorentz-symmetric design boost network performance in jet physics?
Phys. Rev. D 109, 056003 (2024) [Crossref] -
Hammad et al., Multi-scale cross-attention transformer encoder for event classification
J. High Energ. Phys. 2024, 144 (2024) [Crossref] -
Diefenbacher et al., CapsNets continuing the convolutional quest
SciPost Phys. 8, 023 (2020) [Crossref] -
Ahmed et al., Application of deep learning in top pair and single top quark production at the LHC
Eur. Phys. J. Plus 138, 795 (2023) [Crossref]
Ontology / Topics
See full Ontology or Topics database.Authors / Affiliations: mappings to Contributors and Organizations
See all Organizations.- 1 Anja Butter,
- 2 3 Gregor Kasieczka,
- 1 Tilman Plehn,
- 1 4 Michael Russell
- 1 Ruprecht-Karls-Universität Heidelberg / Heidelberg University
- 2 Eidgenössische Technische Hochschule Zürich / Swiss Federal Institute of Technology in Zurich (ETH) [ETH Zurich]
- 3 Centro Svizzero di Calcolo Scientifico / Swiss National Supercomputing Centre [CSCS]
- 4 University of Glasgow
Funders for the research work leading to this publication