Neural network-based approach to phase space integration
Matthew D. Klimek, Maxim Perelstein
SciPost Phys. 9, 053 (2020) · published 19 October 2020
- doi: 10.21468/SciPostPhys.9.4.053
- Submissions/Reports
-
Abstract
Monte Carlo methods are widely used in particle physics to integrate and sample probability distributions (differential cross sections or decay rates) on multi-dimensional phase spaces. We present a Neural Network (NN) algorithm optimized to perform this task. The algorithm has been applied to several examples of direct relevance for particle physics, including situations with non-trivial features such as sharp resonances and soft/collinear enhancements. Excellent performance has been demonstrated in all examples, with the properly trained NN achieving unweighting efficiencies of between 30% and 75%. In contrast to traditional Monte Carlo algorithms such as VEGAS, the NN-based approach does not require that the phase space coordinates be aligned with resonant or other features in the cross section.
Cited by 45
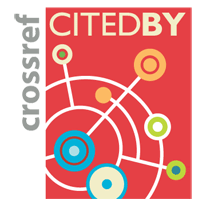
Authors / Affiliations: mappings to Contributors and Organizations
See all Organizations.- 1 2 Matthew Klimek,
- 1 Maxim Perelstein
- National Science Foundation [NSF]
- Samsung Science and Technology Foundation (through Organization: 삼성 / Samsung (South Korea))