Extracting electronic many-body correlations from local measurements with artificial neural networks
Faluke Aikebaier, Teemu Ojanen, Jose L. Lado
SciPost Phys. Core 6, 030 (2023) · published 17 April 2023
- doi: 10.21468/SciPostPhysCore.6.2.030
- Submissions/Reports
-
Abstract
The characterization of many-body correlations provides a powerful tool for analyzing correlated quantum materials. However, experimental extraction of quantum entanglement in correlated electronic systems remains an open problem in practice. In particular, the correlation entropy quantifies the strength of quantum correlations in interacting electronic systems, yet it requires measuring all the single-particle correlators of a macroscopic sample. To circumvent this bottleneck, we introduce a strategy to obtain the correlation entropy of electronic systems solely from a set of local measurements. We show that, by combining local particle-particle and density-density correlations with a neural-network algorithm, the correlation entropy can be predicted accurately. Specifically, we show that for a generalized interacting fermionic model, our algorithm yields an accurate prediction of the correlation entropy from a set of noisy local correlators. Our work shows that the correlation entropy in interacting electron systems can be reconstructed from local measurements, providing a starting point to experimentally extract many-body correlations with local probes.
Cited by 7
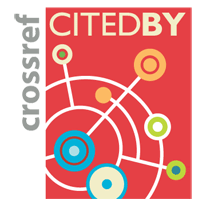
Authors / Affiliations: mappings to Contributors and Organizations
See all Organizations.- 1 2 3 Faluke Aikebaier,
- 2 3 Teemu Ojanen,
- 1 Jose L. Lado
- 1 Aalto-Universitetet / Aalto University
- 2 Helsinki Institute of Physics
- 3 Tampereen Yliopisto / Tampere University