The NFLikelihood: An unsupervised DNNLikelihood from normalizing flows
Humberto Reyes-González, Riccardo Torre
SciPost Phys. Core 7, 048 (2024) · published 31 July 2024
- doi: 10.21468/SciPostPhysCore.7.3.048
- Submissions/Reports
-
Abstract
We propose the NFLikelihood, an unsupervised version, based on Normalizing Flows, of the DNNLikelihood proposed in [Eur. Phys. J. C 80, 664 (2020)]. We show, through realistic examples, how Autoregressive Flows, based on affine and rational quadratic spline bijectors, are able to learn complicated high-dimensional Likelihoods arising in High Energy Physics (HEP) analyses. We focus on a toy LHC analysis example already considered in the literature and on two Effective Field Theory fits of flavor and electroweak observables, whose samples have been obtained through the HEPFit code. We discuss advantages and disadvantages of the unsupervised approach with respect to the supervised one and discuss a possible interplay between the two.
Cited by 2
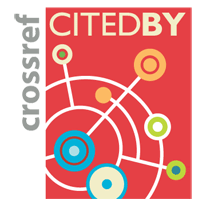
Authors / Affiliations: mappings to Contributors and Organizations
See all Organizations.- 1 2 3 Humberto Reyes-González,
- 3 Riccardo Torre
- 1 Rheinisch-Westfälische Technische Hochschule Aachen / RWTH Aachen University [RWTH]
- 2 Università degli Studi di Genova / University of Genoa [UniGe]
- 3 Istituto Nazionale di Fisica Nucleare Sezione di Genova / National Institute of Nuclear Physics Genoa Section [INFN Genova]
- Deutsche Forschungsgemeinschaft / German Research FoundationDeutsche Forschungsgemeinschaft [DFG]
- Ministero dell’Istruzione, dell’Università e della Ricerca (MIUR) (through Organization: Ministero dell'Istruzione, dell'Università e della Ricerca / Ministry of Education, Universities and Research [MIUR])