Codebase release r1.4 for CoVVVR
Prasanth Shyamsundar, Jacob L. Scott, Stephen Mrenna, Konstantin T. Matchev, Kyoungchul Kong
SciPost Phys. Codebases 28-r1.4 (2024) · published 4 March 2024
- doi: 10.21468/SciPostPhysCodeb.28-r1.4
- publication repo
- live repo (external)
- Submissions/Reports
-
This Publication is part of a bundle
When citing, cite all relevant items (e.g. for a Codebase, cite both the article and the release you used).
DOI | Type | |
---|---|---|
10.21468/SciPostPhysCodeb.28 | Article | |
10.21468/SciPostPhysCodeb.28-r1.4 | Codebase release |
Abstract
Monte Carlo (MC) integration is an important calculational technique in the physical sciences. Practical considerations require that the calculations are performed as accurately as possible for a given set of computational resources. To improve the accuracy of MC integration, a number of useful variance reduction algorithms have been developed, including importance sampling and control variates. In this work, we demonstrate how these two methods can be applied simultaneously, thus combining their benefits. We provide a python wrapper, named CoVVVR, which implements our approach in the VEGAS program. The improvements are quantified with several benchmark examples from the literature.
Cited by 1
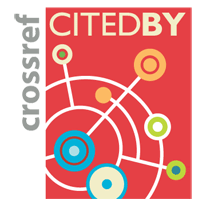
Authors / Affiliations: mappings to Contributors and Organizations
See all Organizations.- 1 Prasanth Shyamsundar,
- 2 Jacob L. Scott,
- 1 Stephen Mrenna,
- 3 Konstantin T. Matchev,
- 2 Kyoungchul Kong
- 1 Fermi National Accelerator Laboratory [Fermilab]
- 2 University of Kansas [KU]
- 3 University of Florida [UF]