Loop amplitudes from precision networks
Simon Badger, Anja Butter, Michel Luchmann, Sebastian Pitz, Tilman Plehn
SciPost Phys. Core 6, 034 (2023) · published 25 April 2023
- doi: 10.21468/SciPostPhysCore.6.2.034
- Submissions/Reports
-
Abstract
Evaluating loop amplitudes is a time-consuming part of LHC event generation. For di-photon production with jets we show that simple, Bayesian networks can learn such amplitudes and model their uncertainties reliably. A boosted training of the Bayesian network further improves the uncertainty estimate and the network precision in critical phase space regions. In general, boosted network training of Bayesian networks allows us to move between fit-like and interpolation-like regimes of network training.
Cited by 12
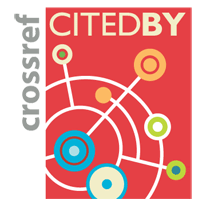
Authors / Affiliations: mappings to Contributors and Organizations
See all Organizations.- 1 Simon Badger,
- 2 3 Anja Butter,
- 3 Michel Luchmann,
- 3 Sebastian Pitz,
- 3 Tilman Plehn
- 1 Università degli Studi di Torino / University of Turin [UNITO]
- 2 Sorbonne Université / Sorbonne University
- 3 Heidelberger Institut für Theoretische Studien / Heidelberg Institute for Theoretical Studies [HITS]
Funders for the research work leading to this publication
- Deutsche Forschungsgemeinschaft / German Research FoundationDeutsche Forschungsgemeinschaft [DFG]
- Horizon 2020 (through Organization: European Commission [EC])